
Precision Ag
Mapping variability
Variable rate nitrogen applications have the potential to save money and improve crop yields. But what is the best way to come up with variable rate management zones that provide economic benefits to the farmer? Could soil sensor maps be a practical data source for identifying meaningful management zones? Those are some of the questions Alberta researchers are answering through a major on-farm precision agriculture study.
The idea for this study was sparked a few years ago when Ken Coles, general manager of Farming Smarter, saw some electrical conductivity (EC) sensors at a precision agriculture conference. He was intrigued by the possibility of using these sensors as an alternative to grid soil sampling for mapping in-field soil variability. “The idea is that we can’t do grid soil sampling to the level of accuracy needed to manage variable rate inputs effectively, plus soil sampling is expensive. So if we can run a soil sensor over a field and get the same or better information, then maybe there is value in it,” he says.
Lewis Baarda, GIS analyst with Farming Smarter, compares the two approaches. He explains that a grid soil sampling system with one sample every five acres would provide 32 data points for a quarter section, and the lab analysis for nitrogen, phosphorus, potassium and sulphur would cost about $1,600. An EC sensor service could produce an EC map of a quarter section with about 50,000 data points for a cost of about $880. EC data tend to be good at predicting soil texture and soil moisture content.
But Coles wanted to do more than compare EC sensor maps and grid soil sampling maps for creating management zones; he wanted to evaluate if those zones were actually meaningful and useful for variable rate management. He says, “Creating management zones based on soil information and then creating a prescription map is not that hard. The challenging part is verifying whether your variable rate management is actually paying for itself. That is really what I wanted to do with this study.”
Coles also wanted to do the study as on-farm research, which added another level of variability. He notes, “Just finding the right co-operators to work with is challenging, and even when we have the right people, we still have human error issues or lack of priority issues. So, not only are we going into a complex environment where we have no control over the variables, but we are literally studying variability and we also have human and equipment and scale variability.”
Starting in 2012, he teamed up with Baarda and Muhammad (Adil) Akbar, precision agriculture specialist and research director with Farming Smarter, to conduct the study on 10 farm fields. The fields are located in southern Alberta, the Drumheller area and the Peace Region (in co-operation with the Smoky Applied Research and Demonstration Association).
Because of the study’s complex objectives, quite a few steps were required in the data collection and analyses for each field, including: conducting soil sensor mapping and grid soil sampling; determining how strongly the EC maps matched up with the soil sample data, yield maps and other data sources; delineating field zones based on these different data sources; conducting a nitrogen fertilizer rate/yield response trial; determining which zone map best predicted yield variability across the field; and determining which zone map provided the best basis for variable rate nitrogen applications.
With funding from Alberta’s Agricultural Initiatives Program, Farming Smarter was able to purchase two EC sensors: the EM38-MK2 and the Veris MSP3. The researchers hooked together the two sensors and pulled them across each field, using Farming Smarter’s onboard RTX-DGPS sensor for georeferencing and elevation recording.
“The EM38 has been around for a long time; they used to use it to map salinity and it’s quite effective for that,” Coles says. The EM38 does not require direct contact with the soil to take EC readings. It is pulled over the field’s surface and takes measurements every few seconds. It can measure EC at depths of 0.75 and 1.5 metres at the same time.
The Veris MSP3 is a mobile sensor platform with three sensors: EC, pH and organic matter. Its EC sensor requires soil contact so it has coulters that maintain soil contact as the equipment is pulled across the field. Like the EM38, this sensor measures EC at both 0.75 and 1.5 metres deep.
The research team scanned each field twice with the two EC sensors, usually in the spring and the fall.
The Veris organic matter sensor measures the soil’s optical reflectance, basically how dark or light the soil is, and those reflectance data are converted to organic matter content by Veris. The pH sensor directly measures soil pH using an on-the-go chemical test, taking a soil sample, testing it and then taking the next sample, while the Veris moves across the field. The pH and organic matter sensors provide fewer data points per field than the 50,000 points generated by the EC sensors.
Soil sampling followed a five-acre grid, with 32 samples for each 160-acre field. The samples were analyzed for nitrogen, phosphorus, potassium, sulphur, organic matter, pH, EC, moisture content and texture.
The co-operators provided yield data collected by their on-combine yield monitors. Baarda notes, “Although the standard practice is to use at least three to five years of yield maps to define productivity zones, in most cases it was a challenge to gather even three years with good spatial coverage.”
Coles adds, “Finding good yield map data is really difficult. There are many reasons for that. One reason is that people don’t save the data; they don’t take the time to transfer it to their computer. Another reason may be that they have two or three combines on the field at the same time, which makes it challenging to stitch the data together. Or it could be they didn’t calibrate it properly.”
For each field, the researchers created zone maps using five different data sources: EC sensor data; historical yield data from the co-operator; grid soil sample data; a visual depiction of the field’s main terrain features; and a composite of yield and EC sensor data. This composite method was included because an objective procedure called principal component analysis identified EC and yield as the two variables, among all the data collected, that best accounted for spatial variability in the 10 fields.
At each field, they conducted a replicated, randomized nitrogen fertilizer rate/yield response trial. The nitrogen fertilizer was applied at seeding. The specific nitrogen rates used in each trial depended in part on what the cooperating farmer wanted to do; usually fewer than five rates were used. The researchers measured the variations in grain yield response and determined the nitrogen rate/yield response curves.
Next, they laid each zone map over the yield response results and determined which of the five zone delineation methods worked best for predicting in-field yield variations and for predicting zones for variable rate nitrogen applications.
Highlights of results
As you can probably imagine, the study involved huge amounts of data that required complex analysis. Akbar, Baarda and Coles are currently finalizing the study’s report, and they hope to also publish some scientific papers.
In terms of the performance of the EC sensors, Baarda says, “Our EC data from the Veris and the EM38 were highly consistent with each other. Also, the spring EC map was always highly consistent with the fall EC map. We could almost take one EC layer and say that’s what the EC map is [for the field] because those patterns don’t change over time and they don’t change between the sensors.” The researchers also found that the EM38 was easier and
less costly to use than the Veris for mapping EC.
Overall, the EC sensor data tended to be strongly correlated with the soil sample data for sand and clay content and soil moisture content, although the strength of the correlations varied from field to field. So, EC sensor maps can give farmers a better understanding of the soil variability in their fields.
However, the EC sensor maps didn’t necessarily predict the spatial patterns in some of the other soil sample data, like nitrogen (N), phosphorus (P), potassium (K), sulphur (S), pH and organic matter. According to Baarda, scale issues could be a factor in the weakness of some of these correlations.
“We’re comparing about 30 data points from soil sampling to about 50,000 from the EC sensors. The [weaker] relationships can get obscured because of the different scales of the datasets. So, even though we don’t see a relationship to N, P, K and S, we can’t necessarily say that those macronutrients don’t correlate to EC. But we get a sense that they probably don’t correlate as strongly as we’d need to make a management response to them.” So the EC sensor maps are not a reliable way to directly estimate variable nutrient rates.
The study also showed grain yield could not be predicted directly from just the EC sensor maps. The correlations with yield were weak or did not exist. Various factors might have contributed to these poor correlations, including the challenges in obtaining good yield data.
Another key finding was the surprising amount of year-to-year variation in the yield patterns. “I think people have a sense that yield patterns are more static than they actually are. Some parts of those spatial patterns are consistent, but statistically those patterns change more than I would have thought,” Baarda says. “So it’s important to have at least three to five years of yield data; the more years you have, the more it helps to balance out the
outlier years.”
The strength of the correlations among the various other data layers – such as elevation, yield, soil nutrients, soil texture, the pH sensor and the organic matter sensor – also varied from field to field.
Because of the field-to-field differences, the different zone delineation techniques had different levels of success depending on the field.
For predicting yield potential, the composite method – pairing up yield and EC sensor data – was the best of the five methods for delineating zones. “In 100 per cent of the instances, the composite method was the most successful in differentiating within-field zones of different yield potentials. So the zones created by pairing EC and yield were meaningful: they predicted where we would have high and low productivity based on the information we had before the growing season,” Baarda explains. “The other four delineation methods failed in differentiating any productivity zones in 20 to 30 per cent of the instances and had varying combinations of complete and/or partial success in the remaining instances.”
He adds, “Some of the composite method’s success is likely due to the use of multiple variables – hedging our bets so to speak. Some of its success also likely lies in the fact that we objectively identified yield and EC as key variables for zone delineation.”
None of the delineation methods were very successful in identifying zones that could be managed differently for
nitrogen in ways that would benefit the farmers economically.
According to Coles, the next step in this research would be to add more layers of data to the analysis, such as remote sensing data from satellites and data from other in-field sensors.
Some take-home messages
“Our big message is there is no single data layer that can be guaranteed to tell you what you need to know to variably manage inputs,” Baarda says. He emphasizes that zone management is a process – each field is unique and you have to be prepared to invest some time in understanding the field’s variability and figuring out what works best for that particular field.
If you’re interested in experimenting with variable rate applications, Coles recommends starting with just a few layers of data.
Baarda thinks an EC sensor map could be a good option for one of those layers. “Not only is EC mapping cheaper than grid soil sampling, but it has a longer ‘shelf life.’ In our experience, EC doesn’t tend to change over time, so a field could be mapped for EC once, and in most circumstances, that data would be relevant for a number of years.” Although the sensors don’t provide the data on nutrient levels that you can get from soil sample analysis, the sensor maps do indicate variations in other soil properties, especially soil texture.
If you want to use your yield monitor data in identifying management zones, then try to ensure the reliability of that data. For instance, be sure to download the yield data from your combine and save it so you can accumulate as many years of data as possible. If you’re using two separate combines on the same field, then consider calibrating them in the same way. If you’re calculating the average yield pattern for a field based on several years of data, exclude any years where the data are skewed because of some external factor, like hail damage on half of the field.
And no matter what data layers you use and what zone delineation method you test, Coles suggests that your on-farm study design should include the steps needed to allow an objective evaluation of whether or not your approach is actually helping you economically.
Coles concludes, “There are lots of people doing variable rate agriculture but very few who are effectively testing and verifying the success.” He adds, “More academic work in this area is sorely needed.”
The study was funded by the Alberta Canola Producers Commission, Alberta Barley and Farming Smarter.
March 7, 2016 By Carolyn King
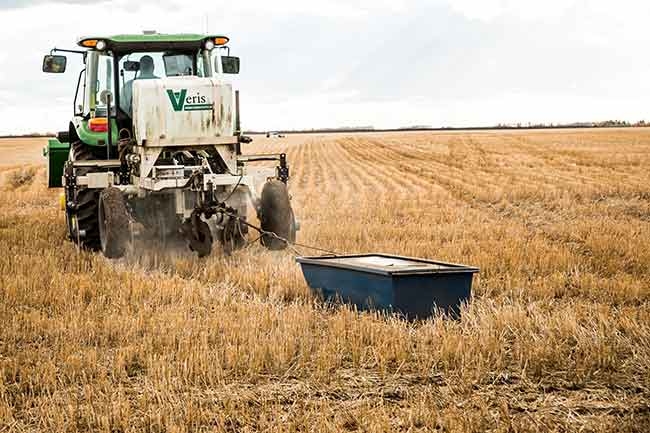